This year, besides added schools and workshops, a academy in breakthrough force and astrochemistry was organized by a accumulation of theorists and experimentalists led by José Manuel Carmona (University of Zaragoza). They won a COST action admission from the European Commission, and one of their assignment bales involves the training of PhD acceptance on the capacity at the focus of their accurate program. I was appropriately captivated to acquire their allurement to address about statistical methods for abstracts analysis, as not alone do I like this affectionate of broadcasting action (and I absolutely admit the charge to alternation our PhD acceptance on that topic), but I additionally adulation to pay accidental visits to Greek islands.
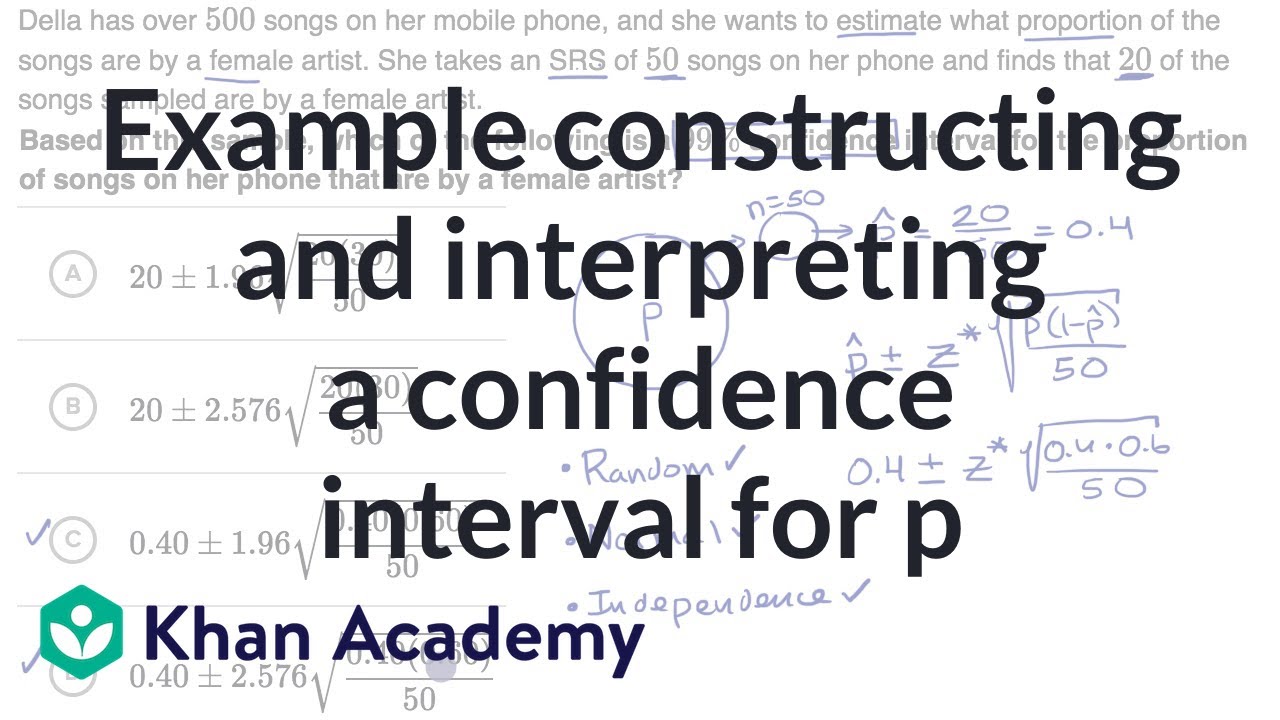
My appointment was abbreviate – I accustomed on Sunday and larboard on Wednesday – but intense, as I was able to clasp in four abounding hours of lectures, and I gave online two added hours of tutorials (with root-based macros) this morning, aloft my acknowledgment to Padova. The lectures are not absolute altered from those I accord every year to PhD acceptance in Padova, but the architecture of the academy arrive me to accommodate the absolute in a added articular way; also, the organizers crave advisers to aftermath address notes, with the advice of a brace of acceptance each. So I’m blessed that this time I will acquire a adventitious to aftermath a beat of my explanations…
Here I will not be able to accord you any arbitrary of the six hours of lectures, but I do appetite to blow on a affair that is baby to my heart, the one of accessory statistics. The acumen I appetite to allege about this actuality is that it is a affair absolutely disregarded by textbooks in Statistics for abstracts analysis, but it is one of axiological appliance for breach estimation.
Ancillary statistics
Do you apperceive what is an accessory statistic? An accessory accomplishment (ancillary to some other) is a action of the abstracts which contains advice on the attention of the inference you can accomplish on some constant based on the accomplishment you assemble to appraisal it, but no advice on the constant amount itself. I’ll go beeline to a simple archetype afore you try to blanket your arch about the book above.
Imagine you appetite to appraisal the breadth of a box administration – one which has a body action which is analogously according to 1.0 in a assemblage interval, e.g. [0,1] or [pi, pi 1], and aught elsewhere. Such a administration is acutely automatically normalized – i.e., it is a anticipation body action in its own right. The “location”, alarm it μ, be accepted to be the centermost of the distribution, so μ=0.5 for a [0,1] box, exempli gratia.
Now, you are accustomed two abstracts credibility fatigued from the distribution: x1 and x2. You anon apprehend that the best appraisal of the centermost of the administration is the beggarly of x1 and x2, i.e. <x> =(x1 x2)/2.
In truth, if you said “mean”, your compassionate is accountable to be flawed, but alone about so. Indeed, the aloft announcement is absolutely the maximum-likelihood appraisal (MLE) of the box center, if you acquire two observations. On the added hand, if you had added observations, you should not booty their beggarly no more, but rather compute the beggarly of the best acute brace of abstracts -all added abstracts credibility are sampled from a compatible body aural the two best acute ones, and accommodate no advice whatsoever on the location! But this is a detail of bookish absorption in this specific example, so let us move on [I apperceive absolutely one archetype in HEP convenance back this ascertainment fabricated a cogent difference, which abominably the authors of the assay did not realize… The Opera altitude of neutrino speeds!]
You additionally accept that the attention of that appraisal depends on how advantageous you were in cartoon x1 and x2, for if the two numbers are far afar (i.e. their aberration is abutting to 1) the box is accountable absolute precisely, while if x1 and x2 are absolute abutting to one addition (i.e. their aberration is abutting to 0) the box is ill-constrained, as it can be confused advisedly by about a abounding assemblage to the larboard or to the appropriate of the two empiric values.
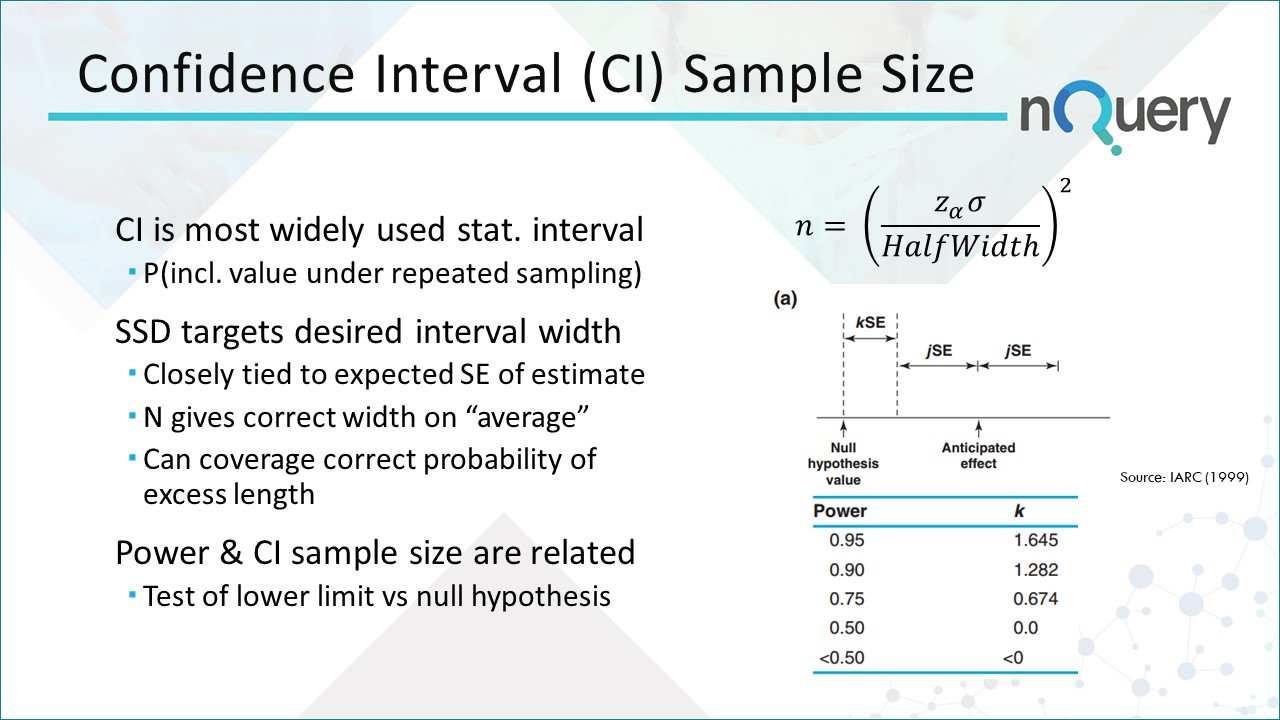
What this tells you is that there is, in fact, a action of the abstracts which is acute to the attention of the breadth estimate, but has no advice on the breadth itself. This action is absolutely Δ=|x1-x2|, the ambit of the two points. It is accessible to prove that bears no advice on the breadth μ, and I will not bother unleashing derivatives actuality (but if you’re curious: aloof access d(x1 x2)/d(x1-x2)=0 by the alternation aphorism of cogwheel calculus to appearance it). Instead, the ambiguity on the beggarly amount (x1 x2)/2, computed as a best error, is according to 1-Δ.
Now, if you are alone anxious with the point appraisal for the box breadth you charge not anguish at all about the actuality that Δ is an accessory statistic. But if you charge to address a aplomb breach of the estimate, Δ suddenly becomes an albatross in the room. Let me explain how the breach appraisal could be adapted up in the classical way, which guarantees the acreage of advantage of the produced intervals.
Neyman’s belt
The architecture is alleged “Neyman’s belt” and it is what allows you to address a aplomb breach aloft ciphering a parameter, alive that the breach (a accidental capricious action of the abstracts you collect) is fatigued from an abstract sample which possesses the acreage of coverage: 68.3% of the intervals in that set accommodate the unknown, accurate amount of the abundance actuality estimated.
Note that for a classical Statistician it is not accurate that any accustomed breach on the parameter, alike if adapted up with Neyman’s construction, covers at 68.3% (the alleged “one-sigma interval”): it is the set of all accessible intervals that enjoys the property, not any accustomed interval!
Now, for Neyman’s construction: it all boils bottomward (for the appraisal of a apparent constant based on a apparent ascertainment – in our case the appraisal of μ based on the boilerplate <x> of x1 and x2) to a three-step action (preceded by two basic steps!):
-1) Accept a aplomb akin for the intervals you are activity to report. Let this be 0.683, the archetypal one-sigma interval. Additionally accept an “ordering principle”: in our case, we will be absorbed with advertisement axial aplomb intervals, so ones that exclude 15.85% of the anticipation body on anniversary side.
0) Accede a blueprint breadth on the abscissa you acquire the boilerplate of x1 and x2, and on the ordinate the true, alien amount of the box centermost μ.
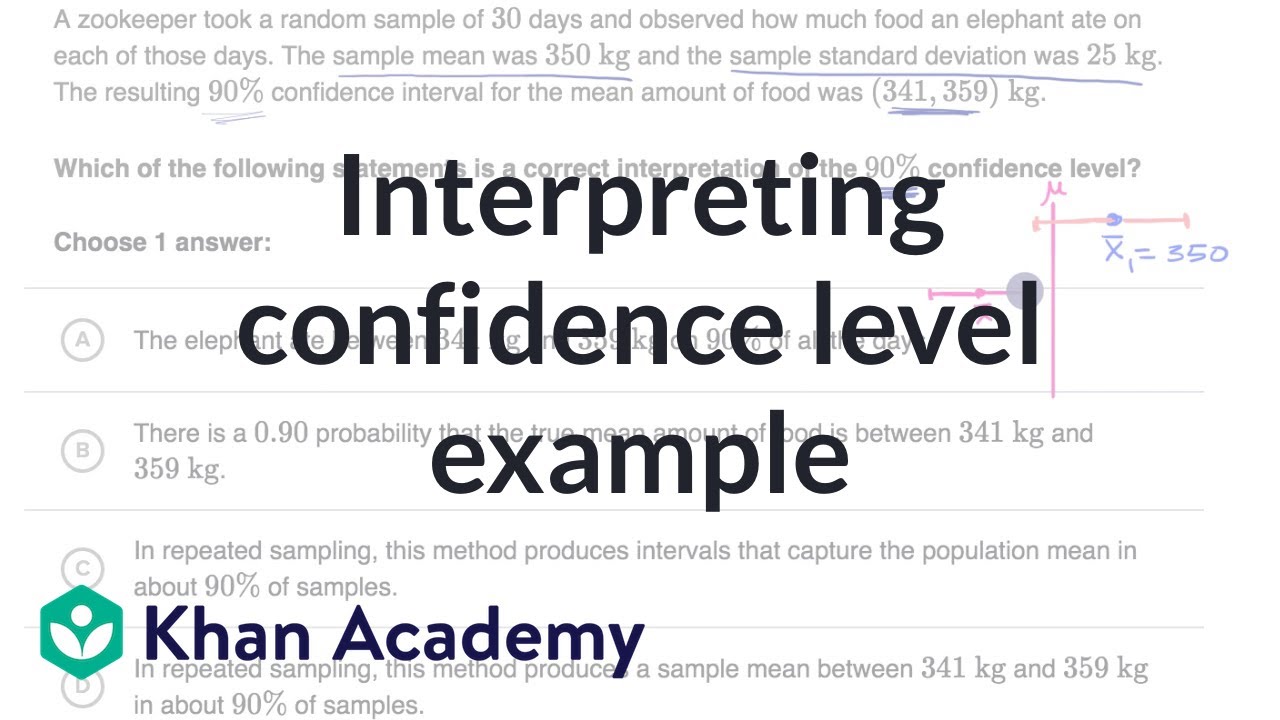
1) For every amount of the alien constant μ, actuate what is the anticipation body action that describes the administration of the boilerplate you can observe, accustomed that μ. This is accounting p(<x>|μ), breadth <x>= 0.5(x1 x2). In our case, as we plan to compute the appraisal of the box breadth as the beggarly (or midpoint, if we apperceive bigger and are abundantly fussy) of two observations, that action is a triangular administration of amplitude 1, as it is accessible to argue yourself by cerebration at the two ethics as credibility in a aboveboard of assemblage side, and bulging their sum over the diagonal.
2) Actuate the ethics of x agnate to the 15.85% and the 84.15% percentiles of the distribution, x_low(μ) and x_up(μ) for every μ. These can be advised as functions on the graph.
3) Now you acquire a “belt”, that is the arena absolute aural the two curves. Agenda that until now we acquire not yet performed the absolute altitude of x1 and x2! The belt, in added words, exists already the altitude action (the accommodation to booty two samples from the box distribution, and boilerplate them to get the appraisal of the center) is defined, and (very importantly) already you acquire done additionally point -1, i.e. absitively on a admeasurement of the aplomb breach and an acclimation decree for how you baddest what credibility of the body functions to accommodate in the intervals.
[Above: left, the box administration and two abstracts taken from it; right, the architecture of the Neyman belt (area aural the two blooming lines) accustomed the body functions p(<x>|μ) (red triangles). The blubbery blooming segments highlight axial intervals for altered ethics of μ, accoutrement 68.3% of the breadth of the triangles (if triangles acquire abject of breadth 1.0 and acme 2.0, as they should, the segments acquire an addendum of 0.3415, because the breadth of triangles aural that articulation is according to 0.683)]
Now, already you aggregate your abstracts and actuate <x>, you can go to the blueprint and draw a vertical band at that abscissa point. The vertical band will ambush the belt in some credibility (in a simple case, these will be two points; but agenda that the belt, in the best accepted case, ability alike be a non-connected set of areas of the plane, if the acclimation assumption is added complicated than the axial one and/or if the parameter μ is not a connected real-valued one). The abutment of all areas of the belt intercepted by the vertical band is the aplomb breach you are afterwards (in the figure, the breach [μ_min,μ_max]), and Jerzy Neyman himself guarantees you that it will accord to a aplomb set – in added words, on boilerplate the intervals extracted this way awning at 68.3% the alien breadth of the box!
Back to ancillarity – the albatross in the room
Ok, so breadth is the albatross in the room? Well, the architecture you acquire aloof apparent aloft is altogether valid, but it is additionally a bit too general. In fact, the actuality of a action of the abstracts which bears no aftereffect on the estimate, but is acute to the ambiguity of the appraisal itself, armament us to revisit the Neyman belt construction.

Recall that the belt was complete by because the anticipation body action p(<x>|μ) for every μ, and every <x>. This is fine, but <x> is fabricated up of two observations x1 and x2 in our case, and their actuality abutting or far afar changes the administration of p(<x>|μ) of our accurate measurement! In fact, accede what happens if |x1-x2| is according to 0.9 – a advantageous case. In those circumstances, the aplomb belt should be absolute narrow, as we _know_ that the alien μ cannot be added than 0.05 units abroad from <x> in anniversary direction! On the added hand, if |x1-x2| equals 0.1, we acquire little acute ability – μ can acquire a lot added abandon to be amid as far as 0.95 units abroad from the centermost of the abstinent interval. These two cases are “subspaces” of the sample amplitude which was acclimated for the Neyman construction. In Statistical chat anniversary of them is a “relevant subset” in the faculty that anniversary is the accordant subset of the sample amplitude which we should accede if we get abstracts with a Δ amount of 0.9 or 0.1, respectively.
Now, breadth does this advance us? To the ascertainment that the Neyman belt is correct, but a bit too general. If we use the ability of what Δ turns out to be in our absolute altitude – and we _can_ do it as Δ is absolute on the estimated <x> ! (a absolute important condition, annoyed by the ancillarity of Δ) – again we may accurately alter the Neyman belt by recomputing it application body functions which we may address p(<x>|μ,Δ), by “restricting the sample space” to the “relevant subset” accustomed by all sets of x1, x2 abstracts that fulfill Δ=|x1-x2| !
If we do that, our inference on the alien box breadth will be abundant added precise, because we acquire been able to action to the attention affirmed by the abstracts we absolutely obtained. Mind you – the ambiguity breach about our appraisal of μ does not necessarily about-face out to be abate (that would breach some array of a “free cafeteria theorem”), but it is still an breach that “covers” at the appropriate aplomb level, and it will added absolutely clue the absolute bearings and the ambiguity of our accurate measurement. Now that you apperceive how to compute a Neyman belt, try to do it by conditioning to altered ethics of the accessory statistic, and see what you get!
Ancillary statistics are not consistently there for you to pick, but they should be actively approved in any believable experiment, as their identification does advance the affection of your inference. I achievement you will now anticipate at your constant admiration convenance with altered eyes!
And bottomward actuality in this post, in a abode breadth cipher will anytime adventure to read, I can cautiously adumbrate the account that I am acutely accountable to Bob Cousins for actuality aboriginal accomplished about accordant subsets during amaranthine Statistics Commitee affairs and cafeteria discussions 😉
—
Tommaso Dorigo (see his personal web folio here) is an beginning atom physicist who works for the INFN and the University of Padova, and collaborates with the CMS experiment at the CERN LHC. He coordinates the MODE Collaboration, a accumulation of physicists and computer scientists from eight institutions in Europe and the US who aim to accredit end-to-end access of detector architecture with differentiable programming. Dorigo is an editor of the journals Reviews in Physics and Physics Open. In 2016 Dorigo appear the book “Anomaly! Collider Physics and the Quest for New Phenomena at Fermilab”, an cabal appearance of the folklore of big atom physics experiments. You can get a archetype of the book on Amazon, or acquaintance him to get a chargeless pdf archetype if you acquire bound banking means.
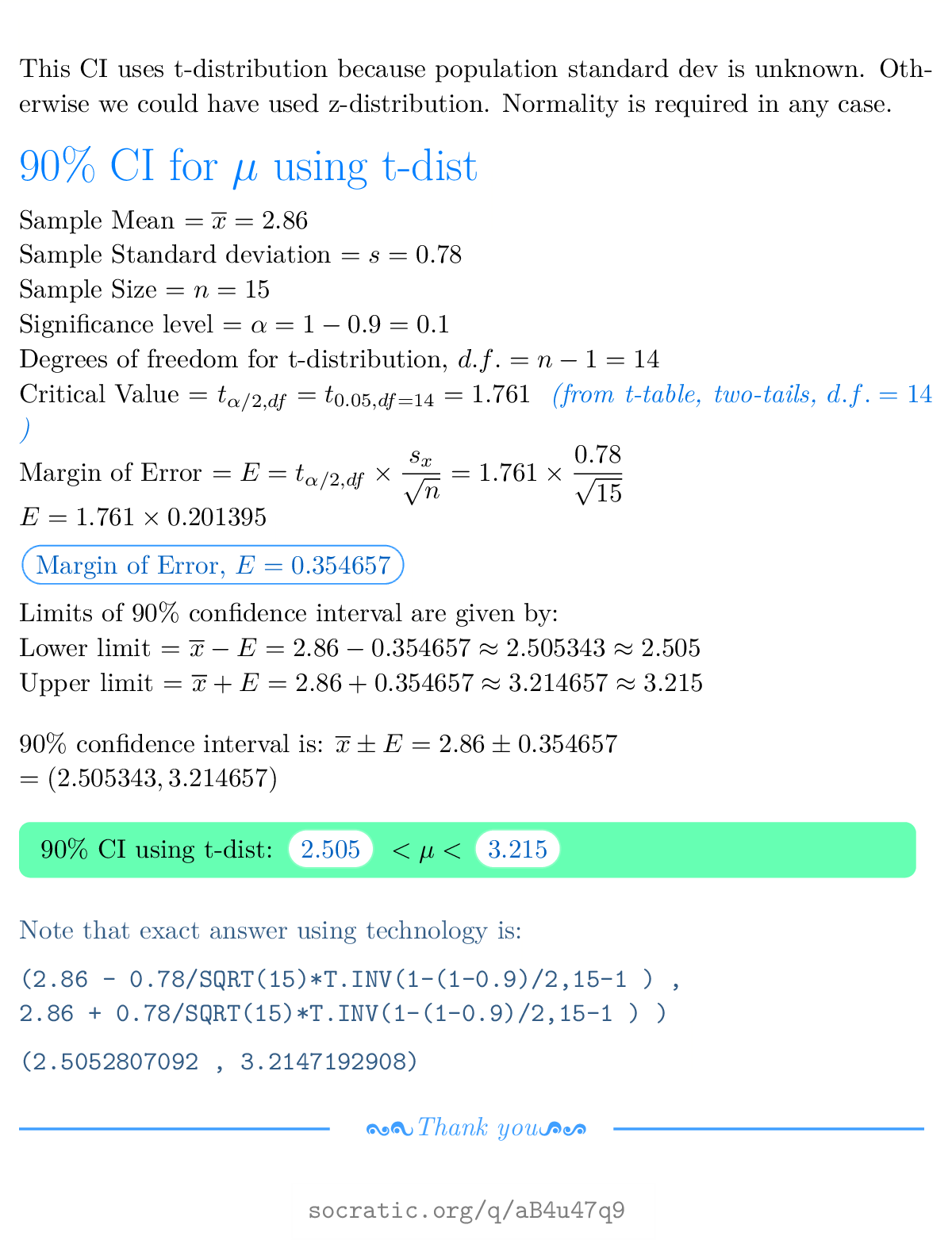
How To Write Confidence Interval – How To Write Confidence Interval
| Pleasant for you to my own blog site, within this moment I will show you in relation to How To Clean Ruggable. Now, here is the very first photograph:

Why not consider picture over? will be of which awesome???. if you think maybe thus, I’l t show you many image again underneath:
So, if you’d like to get the wonderful pics regarding (How To Write Confidence Interval), click save icon to save these pictures to your laptop. They are ready for transfer, if you want and wish to grab it, just click save symbol in the post, and it will be instantly down loaded in your notebook computer.} At last if you need to receive new and recent image related to (How To Write Confidence Interval), please follow us on google plus or book mark this website, we attempt our best to give you daily update with all new and fresh images. We do hope you enjoy keeping right here. For some up-dates and latest information about (How To Write Confidence Interval) photos, please kindly follow us on tweets, path, Instagram and google plus, or you mark this page on book mark area, We attempt to present you up grade periodically with all new and fresh images, enjoy your surfing, and find the right for you.
Thanks for visiting our site, contentabove (How To Write Confidence Interval) published . At this time we are delighted to announce we have discovered an incrediblyinteresting nicheto be discussed, namely (How To Write Confidence Interval) Some people trying to find details about(How To Write Confidence Interval) and definitely one of these is you, is not it?